An advanced control system helps alleviate workflow inefficiencies and reliance on manual adjustments in a complex chemical process
Synthesizing principles of engineering and biology, Solugen Inc. (Houston; www.solugen.com) has developed and commercially deployed a unique carbon-negative platform for peroxide manufacturing. The platform, called Bioforge, employs a renewable plant-based extract and combines it with deionized water and compressed air, which is then processed through an enzyme reactor to continually extract molecules as the reaction is occurring. The intermediate molecules are imbued with more compressed air and poured over Solugen’s metal catalysts to create a final product. For more details on Solugen’s process, see Enzyme Engineering Enables Bio-based Hydrogen Peroxide, Chem. Eng., May 2019, p. 7.
The molecules Solugen produces comprise the foundational building blocks of ingredients in numerous manufacturing sectors, including agriculture, water treatment, oil-and-gas, cleaning products, food additives, personal care and concrete production. Solugen has a location in Slaton, Tex. and a primary manufacturing plant in Houston (Figure 1). As with any new process technology, commercialization and subsequent stable operation involve many technological hurdles. This article describes the implementation of an advanced process-control scheme that enabled Solugen to reduce operational inefficiencies and improve production yield.
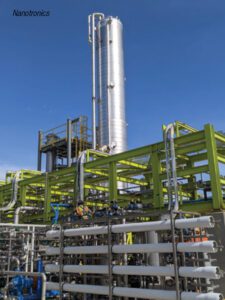
FIGURE 1. At Solugen’s manufacturing plant in Houston, a novel technology uses enzymatic reactors to produce carbon-negative chemical building blocks
Overcome scalability challenges
Like any new process, scalability is a concern. Within Solugen’s process, there is critical variation across the catalysts that are deployed and the chemistry on which the process relies. This variation requires quasi-human interpretation of a given state to successfully progress the process. Through continuous use, the particles become inconsistent in structure and do not perform in agreement with the theoretical ideal. Therefore, operators must be able to adjust plant settings to account for discrepancies in saturation, which can be unreliable when left to human capacity or inference alone. Introducing a deep-learning control strategy to the plant was seen as one potential solution for real-time optimization of such complex objectives. To adjust for variation in particle composition, it is necessary to derive an intelligent control scheme that automatically adapts to process fluctuations in-situ.
Nanotronics (Cuyahoga Falls, Ohio; www.nanotronics.co) was consulted by Solugen to test the applicability of the nControl proprietary process-control platform. The nControl system builds latent representations of critical information using empirical observations in the form of sensor data, extracting features contributing to deviation in normal performance. It is able to surmise latent variables, whose analytical definition is nearly intractable, and provide a rich snapshot of the past, present and future state of the process.
The nControl prediction and control models operate through dimensionality reduction — compressing informationally sparse states, or data extracted from disparate sensors, into a representation that conserves important attributes of the observed phenomenon. This results in higher informational density for both humans and machines to make operational decisions — a key benefit in Solugen’s process, where human interaction is classically the first response to varying process parameters.
Measurements are encoded with nonlinear, time-aware and sensor-agnostic deep networks, ensuring that each transformation embeds additional, pertinent information in its latent representation. These representations are simultaneously fed forward for the ultimate purpose of control via artificial intelligence (AI), but are also interpreted for immediately useful consumption by human observers, in the form of anomaly detection and alerting, as well as quality metrics.
The anomaly detection capabilities nControl offered to Solugen were highly effective in streamlining and refining the site’s chemical processes. The platform is able to adjust for, as well as predict, degradation of capital equipment, unanticipated restriction of the valves and pipes that house feedstocks and atypical chemistry correlated to exothermic events. Inferred quality metrics allow engineers and operators to determine and execute goals in real time by providing immediate feedback on process deviations with acute accuracy (Figure 2).
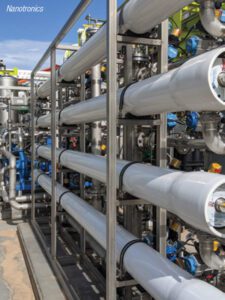
FIGURE 2. The nControl platform provides process adjustments and predictions related to degradation of equipment, such as valves and pipelines, within Solugen’s plant
Solugen’s control strategies benefit from a reduction in the dimensionality of inferred quality metrics that highlight the important features of both human-legible, useful observations, and the values targeted by the control strategy, which is able to act faster and more precisely than a human agent.
Such dynamic performance is unique among control systems for complex chemical processes. Competing platforms operate as backward-looking manufacturing analytics that do not include real-time process control. They may provide retrospective reports and insights or instantaneous monitoring and alerting, but they do not have the capacity to actively control production processes, and no software-only solution is capable of making these interventions. Typically, factory operations must be networked via a programmable logic controller (PLC) to achieve this effect, whereas the nControl platform can act on occurring processes immediately.
Furthermore, nControl is nonparametric, meaning that it learns to recognize new empirical patterns not captured in theoretical formulations of the production process, such as physics-based or chemistry-based simulations. It is also inherently multivariate and retains interactions between sensors. Its multiscale structure enables it to capture periodicities and other patterns at different time scales — predictions can be thought of as a composite of multiple timelines.
For Solugen, the initial models from Nanotronics are unsupervised and do not require labor-intensive data labeling. The system deftly handles datasets with significant variance and can be trained on a variety of operations — startup, shutdown, maintenance and various run conditions — and has successfully predicted processes across diverse situations, including conditions that were not visible in training data. ■
Edited by Mary Page Bailey
Authors
Damas Limoge is the director of applied research at Nanotronics (2251 Front Street, Suites 108-109, Cuyahoga Falls, OH 44221; Phone: 330-926-9809; Email: [email protected]), focusing on nonlinear system control, real-time analysis with generative autoencoders, time-series attention networks, deep reinforcement learning techniques and developing the nControl and AIPC platforms. He holds a M.S. degree in mechanical engineering from the Massachusetts Institute of Technology (MIT) and has previously worked with MIT’s Lincoln Laboratory, Resolute Marine Energy, General Electric and Goodrich in various mechanical engineering and simulation design roles.
Cecilia McLaren is a marketing and communications associate at Nanotronics (Brooklyn Navy Yard Building 20, Brooklyn, NY 11205), where she serves as a writer, editor and media producer. She is the producer of Utility + Function, Nanotronics CEO Matthew Putman’s podcast, which explores an interdisciplinary range of subjects, including AI’s impact on social, economic and political spheres. McLaren holds a B.S. degree in media, culture and communication from New York University, and has been involved with the university’s Digital Theory Lab, a research cohort convening graduate students and faculty members from universities across the globe to conduct multimodal inquiry converging technological artifacts and humanities-based methodologies.
Note: nControl is a registered trademark of Nanotronics