Predictive maintenance for rotating machinery is gaining prominence as plant operators embrace analytics and learn how to approach their operating benchmarks
Rotating machines, such as motors, compressors, pumps and turbines, are critically important components of plant operations, and must perform reliably and efficiently at all times. However, given the high pressures and harsh environments in which these machines operate, equipment failures are all too common.
To keep equipment running for as long as possible, companies must invest in maintenance. Historically, many aspects of these maintenance tasks were less than ideal, but few alternatives existed. In many cases, simple visual inspection was used to determine equipment condition. Often, plant managers ran equipment until failure instead of performing preventive maintenance. This reactive maintenance approach results in unscheduled downtime, higher labor costs and faster deterioration of assets.
In order to avoid these costly scenarios, many companies began to embrace preventive maintenance. With preventive maintenance strategies, statistical trend data and analysis are used to determine the optimal intervals for servicing equipment. The biggest challenge with this approach is that it is time-based. Equipment is inspected and addressed at regular intervals regardless of a truly identified need to do so. It is thus possible to induce failure by tampering with equipment more often than necessary. In some cases, equipment is replaced without assessing its remaining life or current performance. Some of these challenges are being addressed through advanced digital technologies that can better predict and diagnose anomalous equipment behavior (Figure 1). Table 1 summarizes the evolution of maintenance practices typically used in the chemical process industries (CPI). This article examines the benefits of predictive maintenance strategies for rotating machinery and also provides guidance in configuring data-analytics platforms and organizing operational benchmarks for rotating machinery. For more on data analytics, see Placing Data Analytics in the Hands of Engineers.
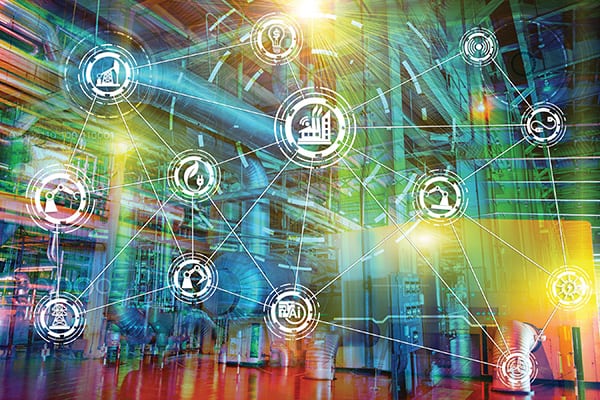
Figure 1. Advances in digitalization and connectivity have helped usher in more proactive maintenance practices
The move to predictive
With vast computational power and the advent of low-cost sensors, preventive maintenance is transitioning into predictive maintenance. Predictive, or condition-based maintenance, as it is also called, identifies issues in equipment before they present serious risks to plant operations or personnel. In a predictive maintenance approach, plant managers use evidence-of-fault to determine how to maintain equipment or even when to replace it. Accurate information on equipment condition enables companies to minimize failure risks, reduce maintenance costs and maximize asset availability.
Critical equipment that can cause a shutdown or other loss of production in the event of a failure are typically outfitted with instrumentation, such as continuous vibration probes, accelerometers, displacement transducers, phase detectors and speed detectors (Figure 2). They also are outfitted with pressure and temperature transducers that measure conditions that change more slowly, such as bearing temperature or oil pressure.
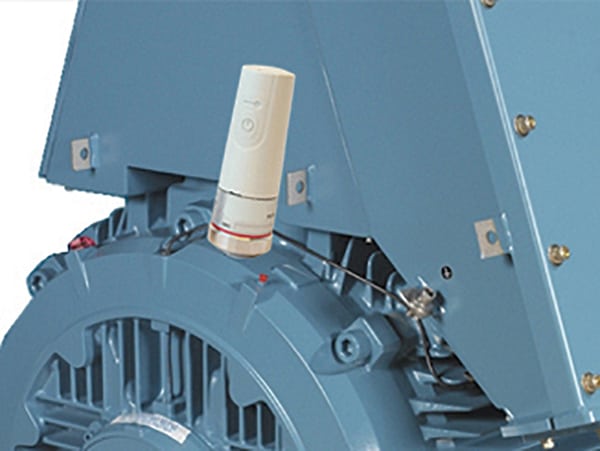
Figure 2. There are many important factors to consider when configuring wired and wireless instruments on different types of rotating machinery
Because bearings are extremely critical components of rotating machines, sensors are frequently installed directly on the bearing to measure vibration and bearing surface temperature. Other sensors are installed on the machine housing to capture overall vibrations, and in the case of electrical motors, to measure critical electrical parameters, such as voltages and currents.
Wired instrumentation also is used in less critical equipment, but this is uneconomical, so monitoring is often limited to high- and medium-criticality machines. Wired sensors allow for continuous machine monitoring, while wireless sensors measure machine status periodically (for instance, once per hour). Wired instruments are better for protecting the machine, while wireless sensors are good for monitoring overall machine condition. Moreover, wired sensors can capture some types of signals and information not achievable wirelessly.
However, unlike in years past, the cost of wireless sensors is decreasing significantly, and they are also becoming easier to install, so they now provide a suitable solution for low-criticality equipment. The most common sensors measure vibrations (accelerometers), surface temperatures and magnetic fields. Prior to wireless technologies, the only way to obtain those signals was to install dedicated probes on the electrical feeding lines, but this is expensive, and therefore, uncommon for low-criticality machines.
Failures lead to downtime
Rotating machines usually operate from 1 to 10,000 rpm and withstand high pressures and temperatures. These factors, combined with centrifugal and centripetal forces, increase wear that will inevitably lead to failure. This could mean several days or weeks of lost production and millions of dollars in downtime and repair costs. To illustrate this point, a risk-assessment study for a gas turbine (Table 2) shows that turbine sub-system failure presents serious risk of significant downtime [ 1]. A 22% failure rate of the compressor, combustion chamber and turbine sub-systems caused an average downtime of 24 hours per failure. But each individual failure could cause 10 to 100 hours of downtime, leading to significant production losses.
Remote monitoring
The most common technologies used in predictive maintenance of rotating machines are infrared thermography, ultrasound analysis, motor-current signature analysis, oil analysis, partial discharge and vibration analysis. All of these methods use evidence-of-fault to determine the maintenance requirements. Table 3 provides details of these technologies.
Although predictive maintenance provides information for timing maintenance activities accurately, it has limitations. Acquiring data using a handheld device (a common practice) is labor-intensive and takes people away from other more productive activities. It is also difficult to gather data from equipment that is in hard-to-reach areas, or areas that pose a danger to the person collecting the data.
However, advances in wireless technology (including a significant drop in deployment costs) and secure wireless communications allow engineers to monitor equipment remotely (Figure 3). Data can be automatically collected even from the most inaccessible sites, and remotely monitored from anywhere in the world.
This represents a great cost-reduction opportunity, as personnel no longer need to spend valuable time physically visiting and interfacing with every asset to gather data. It also has a positive impact on safety, since remote monitoring allows personnel to avoid dangerous areas of the plant.
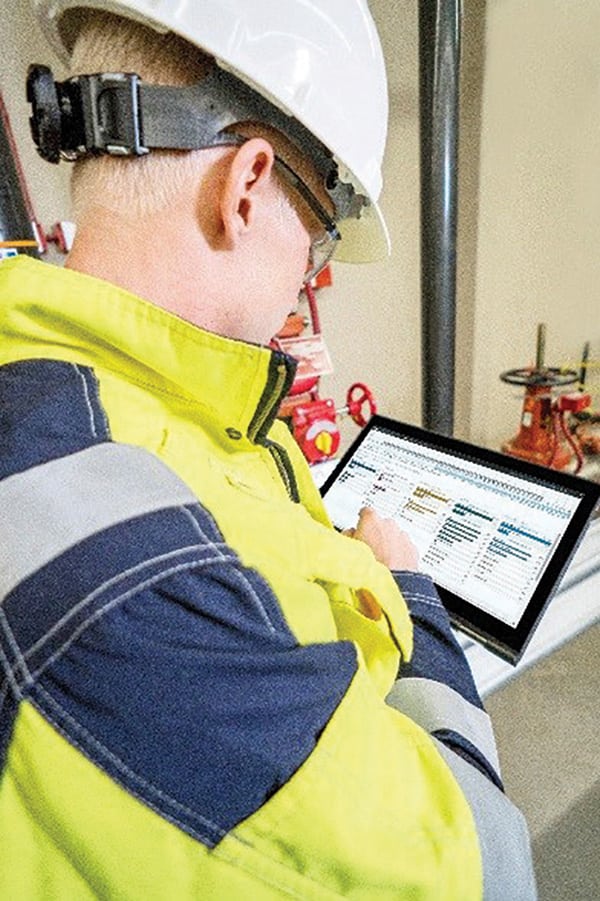
Figure 3. Handheld devices, which enable more effective remote-monitoring programs, are becoming more widely used in the CPI
Data analytics adds power
While remote monitoring is an exciting advancement, robust data collection and analytics make predictive maintenance truly powerful. Data analytics enables the accurate modeling of conditions and factors that lead to equipment failure, thus allowing plant engineers to stay ahead of problems before they happen. The following is a hierarchy for remote monitoring and data analytics that is currently being used in some CPI plants:
- Field level: Sensors that collect basic data, such as current, temperature and torque, on equipment and send it to an onsite edge device. An edge device, such as a router or multiplexer, serves as an entry portal into core networks
- Edge device: Data streams from sensors are aggregated and analyzed by sophisticated software algorithms to identify, categorize and prioritize issues. Plant-wide solutions for condition monitoring are implemented, leading to realtime optimization insights
- Cloud: Data from different sites are aggregated into remote service centers and used by subject matter experts to provide advanced services across sites. This allows for collaboration between sites, headquarters and suppliers, leading to better-informed decision-making. Information is also used to support field services
While deploying remote monitoring is challenging, figuring out what to do with all the data is even more challenging. Suppliers are inundating producers with remotely monitored data, but it can be difficult to extract useful and directive information and present it as easy-to-understand data analytics for appropriate actions. A robust data-analytics platform is necessary to provide users with the insights to resolve issues before they become problems and must be part of a larger network of devices and services (Figure 4). The following questions are among those that will be addressed by a well-designed data-analytics platform:
- What are the key performance indicators (KPIs) that should be monitored for rotating machines?
- How should they be categorized and displayed so that users can quickly turn raw information into actions?
- Which KPIs have the highest impact on equipment and, therefore, process performance?
- How are benchmarks used to calculate ideal performance levels?
- How should deviation from ideal performance be analyzed so that mitigating actions can be implemented?
- What kind of notifications should be sent to alert users when there is a problem?
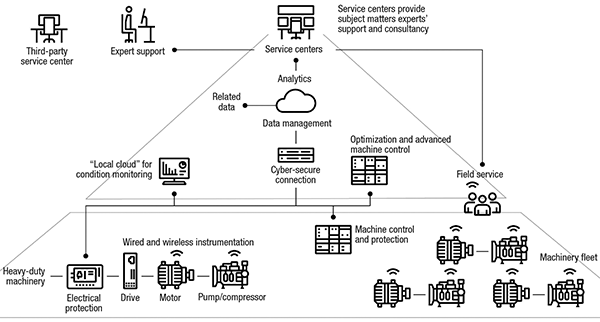
Figure 4. An effective predictive maintenance strategy includes connectivity between many operational levels and devices
These same technologies can be applied beyond rotating machinery to other equipment types. For non-critical assets, predictive analytics capabilities are more often used to re-think maintenance intervals; for example, how often to perform certain maintenance activities based on actual machine usage instead of time-based intervals. Due to the large volume of equipment in a typical CPI plant, these seemingly small changes can prove to be quite valuable. Multiplied over thousands of assets, those savings in maintenance costs quickly become significant. Even more significant is avoiding production downtime. Keeping production running, incremental margins increasing and orders filled are crucial.
Organized KPI data get results
Data-analytics platforms must automatically identify, categorize and prioritize KPIs so that the most important issues by severity and criticality are addressed first by operational decision-makers. Table 4 provides some examples of specific KPIs for rotating equipment.
The goal is to give operators an unambiguous view of which issues require immediate attention, which can be monitored, and which are less important but may require attention at a later time. This way, in a few quick views, operators can see critical issues and make important decisions on how to address them. In this scenario, KPIs are categorized into four groups:
- Process: Information regarding the thermodynamic performance of the process machine
- Mechanical: Information regarding the mechanical condition of rotating equipment
- Electrical: Information regarding the condition of electrical equipment, including auxiliary systems, such as switchgears and transformers
- Control: Information regarding performance of the machine control system, including instrumentation and actuators
What operators should get from these systems is a time-series analysis that shows spikes (such as anti-surge control change between 2:30 and 3:00 pm), indicating high deviation from optimal control limits. This information prompts the operator to look more closely to determine the root cause of the deviation and take necessary action.
Data-analytics systems also should alert plant operations via email or text when KPIs exceed set limits, helping them stay informed of issues even when they are away from the site. This promotes faster resolution of problems, improved equipment condition and higher process performance.
Depending on the type of KPI-triggered alert, several actions can be taken:
- If the KPI can trigger a mechanical or electrical service, such as a repair call, then the maintenance engineer issues a request for field engineers to verify and perform the repair
- If the KPI is related to the control system of the machine, the action is typically to verify the status of the control system and re-tune it
- If the KPI is related to the process performance of the machine, then the action is in the hands of the process engineers and operators, who will decide the best way to ensure the optimal performance of the process surrounding the machine
Establishing a predictive maintenance program for rotating machines requires a systematic and methodical approach that takes into account maintenance strategies that include remote monitoring, robust data analytics and, most importantly, qualified personnel who can turn information into actionable insights that lead to lower costs, improved productivity and higher overall plant performance.
Edited by Mary Page Bailey
References
1. Wassan, R.K., Majid, M.A., Mokhtar A.A., Risk Matrix Model for Rotating Equipment, MATEC Web of Conferences, Vol. 13, No. 03006, 2014.
2. Hashemian, H.M., Wireless Sensors for Predictive Maintenance of Rotating Equipment in Research Reactors, Annals of Nuclear Energy, Vol. 38, pp. 665–680, 2011.
Author
Diego Pareschi is the global product manager for rotating-machinery products and services for the oil, gas and chemical markets at ABB (George Hintzenweg 81, 3068 AX, Rotterdam, the Netherlands; Email: [email protected]). In this role, he manages the portfolio of solutions for gas compressors, pumps, turbines and electrical motors, including the use of digital technologies to ensure predictable, safe and efficient operation. Pareschi joined ABB in 2010 and has held various roles in research and development, product development and marketing in Switzerland, Italy and the Netherlands. He earned an M.S. in automation and control engineering from Politecnico di Milano, Italy.